Insight
Using the digital data landscape to tackle COVID-19 challenges in the developing world
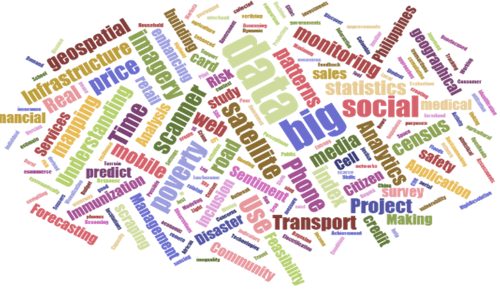
Insight
The NIRAS Data Future’s Hub in partnership with DFID’s Frontier Technologies Hub has been carrying out the Frontier Data Study for the UK’s Department for International Development (DFID) to identify the best emerging data opportunities for DFID and how to best operationalise them.
The full study will be released soon (follow us on LinkedIn for news on its release or our main web page). Our research has identified that there are three categories of new digital data sources that, in terms of the quality of the data they produce, have the most potential to help in international development. With the right approaches to risk management, these can be utilised now to add value in many different contexts:
The Study will provide detailed user guidance for these sources and look in more depth at the strengths of weaknesses of the many options we now have in the new data landscape, including setting out other emerging data opportunities that require some significant developments before they can be confidently used.
Prevention and surveillance
Diagnosis and treatment
Mitigating economic impacts
The Data Futures Hub is also about to launch our Corona Virus Knowledge Hub, bringing together our pick of the global innovations underway and a live dashboard of some of the most authoritative sources of data.
There is no magic wand in using digital data sources. The benefits and risks need to be weighed up, and the risks managed according to each need for data in specific contexts and the characteristics of each data source.
Passive locational data from mobile phones (automatically collected by mobile network operators or from smartphone apps) has huge potential, particularly in combination with EO data. This includes helping reduce the spread of COVID-19 and understanding the economic impacts by understanding when, where, and with whom people move. But it has not yet been used extensively, not least because of privacy concerns. There are signs that governments are willing to relax privacy rules for a range of digital data with the aim of rapidly releasing data for research. While this may help in making data available and ensuring they can be anonymised to protect privacy, the ethical risks need close management. Moreover, a relaxing of the rules may change, at worse increase, the overall risk environment in the long term.
AI-derived data, such as through machine learning, has already been widely used during the pandemic and has provided some useful insights to inform decision-making. As opposed to passive locational data, there is also huge scope to harvest the data that individuals actively input to smartphones and other devices, such as gathering opinions shared on social media and even targeting information tailored to different population groups. But AI-derived data need to be used with caution due to a range of significant data quality and ethical reasons.
The best insights for informing decision-making, in terms of data quality, are likely to come from combining new data sources and combining them with traditional data such as surveys and admin data (such as hospital records). Ethical risks, however, potentially grow with the increase in the volume, interconnectivity, and variety of data held about individuals and their traceability to individuals. The long-term legacy of ill-thought action in this area needs to be carefully considered. Not least in terms of human rights and people’s willingness to share any personal data in the future. Moreover, in developing countries in particular, investments in traditional sources, such as crucial admin data, will need even greater attention as public sector organisations are stretched in dealing with increased demands for their services and data.
While official statistics can be slow, the Data Futures Hub recommends that data innovation should always be seen in the context of official statistics: